This article is one of a five-part series exploring the fundamentals and practical application of artificial intelligence for professionals in the medical aesthetics industry. We are not responsible for any hostile AI takeovers, Armageddon, human extinctions, or cheesy dystopian plots. We will, however, take full credit for any incredible improvements to your marketing program. That we are used to.
Back in 2022, we wrote a one-off piece about How Artificial Intelligence Was Changing Healthcare Marketing. Back then, huge strides were already being made in the practical applications of AI.
That article was lofty, covering a wealth of information about contemporary tools. AI then didn’t even have the buzz it has now, but we knew big developments were on the horizon and that it would be prudent to start getting medical professionals to pay attention to the conversation.
But then, the second half of 2022 hit, and suddenly, the capabilities of AI became much more practical (and accessible) to the average person.
People were generating new social media avatars by the dozen, laughing as their likenesses were put into outer space with ridiculous haircuts or going full #newprofilepic when they looked way cooler by illustration than by daylight.
It didn’t stop there. Art diffusion models like Midjourney, Stable Diffusion, and DALL-E 2 started getting traction as the buzz grew. The tools were, for the most part, fairly intuitive, and they were giving people access to amazing generated images with a few untrained clicks.
This opened up a world of visuals to people who, up to that point, may have only had a stock imagery subscription for their visual needs, if anything at all.
Then, as tools like Jasper, Copy.AI, and other AI writing tools were chasing LinkedIn clout harder than Dom Toretto chases family, OpenAI launched ChatGPT, causing the gasp heard around the world of English teachers collectively anxious at the next step in automation.
This was the arrival of a calculator. For words.

Copywriters loudly lamented their doom — easily convincing themselves that they were the next McDonald’s ordering kiosk.
(On the other hand, humanities students shed gentle happy tears of productivity and easy degrees.)
All of this context paints an astonishing picture: in the last three months, practical artificial intelligence tools exploded in what would be considered a short time — even for technology, which cycles more rapidly than most.
The dust is still settling, and while it turns out we still need artists, copywriters, and English teachers, we have also figured out how these new tools can be an asset used specifically for your medical practice.
Before we dive in, though, we need to lay a foundational understanding so that when we say things like “machine learning” or “natural language processing,” you truly understand what we mean, and aren’t discarding it into a little box named “jargon.”
That’s what this part of our artificial intelligence series will cover.
Artificial Intelligence, Defined
Here is a definition of artificial intelligence that you could potentially submit to Webster’s:
Artificial Intelligence (AI) refers to the simulation of human intelligence processes by machines, especially computer systems. These processes include learning (the acquisition of information and rules for using the information), reasoning (using the rules to reach approximate or definite conclusions), and self-correction.
But Webster's approach doesn’t help you here. You probably already knew it had something to do with computers learning how to process information and appear intelligent in the same manner that a person does.
After all, everyone has seen Terminator, right? Or any of the hundreds of movies that feature AI? Here’s a short list if you have some catching up to do:
What the definition we provided above is ultimately getting at is that artificial intelligence is built on a computer’s ability to do two things: process inhuman levels of information and then use that information to find patterns and predict a desired human outcome.
The data processing part of artificial intelligence has been used for years without concern, mostly because all it does is find patterns and then present its findings to a human for further decision-making. The buzz AI is facing now is because that second bit, the ability to predict the next desired outcome, has become much more usable for the average person.
So, a definition that is more applicable to you and the average user is something along the lines of this:
A super-intelligent program that can deliver a text or an image asset that closely resembles a human's output in a fraction of the time.
Unless you are a tech geek, the details about AI and how they work probably don’t matter to you as much as the actual tools they provide do. Nevertheless, we are here to lay a foundation, and we’ll be damned if we leave any cracks.
Key AI Terminology
This section is pretty straightforward, and, to be completely honest, you don’t really need to read this right now — but you should bookmark it for when you inevitably come across an AI term that you need quickly defined.
Machine Learning (ML) — A method of data analysis that automates analytical model building, based on the idea that systems can learn from data, identify patterns, and make decisions with minimal human intervention.
Deep Learning — A subset of machine learning that uses neural networks with many layers to analyze various factors with a structure similar to the human brain.
Neural Network — A series of algorithms that mimic the operations of a human brain to recognize relationships between vast amounts of data.
Natural Language Processing (NLP) — The ability of a computer program to understand normal human language in a valuable way.
Supervised Learning — A type of machine learning where the model is provided with labeled training data.
Unsupervised Learning — A type of machine learning where the model isn't provided with labeled training data and must find patterns on its own.
Semi-Supervised Learning — A type of machine learning that uses a combination of a small amount of labeled data and a large amount of unlabeled data for training.
Computer Vision — The field of study surrounding how computers can gain high-level understanding from digital images or videos.
Generative Adversarial Network (GAN) — A class of machine learning frameworks where two neural networks contest with each other in a game.
Chatbot — An AI software designed to interact with humans in their natural languages.
Predictive Analytics — The use of data, statistical algorithms, and machine learning techniques to identify the likelihood of future outcomes based on historical data.
Robotic Process Automation (RPA) — The technology that allows anyone today to configure computer software, or a “robot”, to emulate and integrate the actions of a human interacting within digital systems to execute a business process.
Artificial Intelligence, a History
Let’s go back to school for a minute. (Don’t worry, no organic chemistry. All history for now.)
An Early AI Spring
The story of artificial intelligence is as old as computing itself, traceable all the way back to World War II. During this period, British mathematician Alan Turing and his team developed the Bombe machine to decrypt German codes.
(Please see Morten Tyldum’s The Imitation Game (2014) for more context — it’s a movie about Alan Turing that earned a 90% on Rotten Tomatoes and a permanent spot in my heart.)

Essentially, the Bombe machine was used to brute-force its way through thousands of Nazi codes, eventually achieving its goal of decrypting the best coding machine in existence, the Enigma, and giving life to the idea that computers themselves had value.
In 1955, the Dartmouth Summer Research Project, a collaborative effort by leaders in various fields to develop the technology behind “thinking machines,” also known at that time as cybernetics or automata theory, was formally proposed by John McCarthy, Marvin Minsky, Nathaniel Rochester and Claude Shannon. The proposal is credited with introducing the term 'artificial intelligence'.
The proposal from McCarthy is now credited with introducing the world to the term “artificial intelligence” for the first time in history.
The Long AI Winter
The optimism and excitement fostered at the Dartmouth Conference gave way to an era often referred to as the golden years of AI. During this period, funding was plentiful, and early successes such as the Logic Theorist, the first AI program ever developed, spurred a belief that human-level AI was just around the corner.
Unfortunately, the technology behind AI could not keep up with the high expectations it had created for itself, and when AI failed to deliver, disappointment set in and funding was pulled, leading to the first AI Winter in the 1970s.

There was some traction gained in the 80s, but other than pop-culture stories, the development of AI was more or less at a standstill. And look, disco in the 70s and 80s really popped off, can you blame the scientists for taking a break?
Don’t get me wrong — there were still believers like Geoffrey Hinton, a.k.a. the godfather of AI, who would ultimately continue to push AI algorithms into existence, fighting the pull of bangers like Boogie Wonderland and Staying Alive, but, for the most part, scientists didn’t think it was worth the investment.
Summer of AI Superblooms
While AI efforts seemed to come to a halt, the mass digitization of our lives did not. Companies like Microsoft and Apple spent an exorbitant amount of resources developing and marketing devices that would incrementally entangle our lives with digital devices, ranging from PDAs in 1992 to the iPhone 15 Plus Pro Max today.
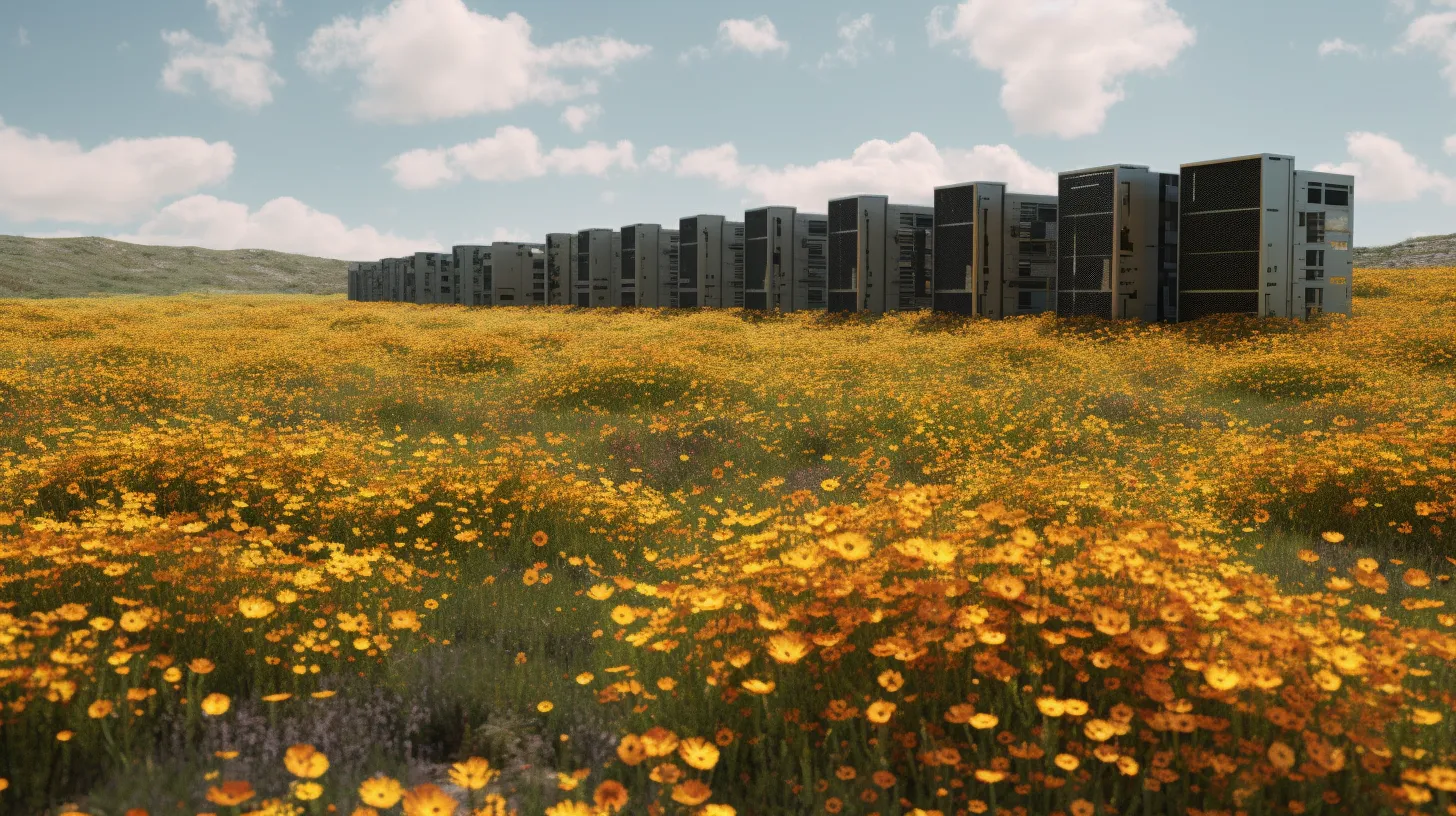
We have all generally been eager to digitize as many aspects of our lives as possible, despite occasional grumblings about how things have progressed.
This is why, in the last decade or so, a lot of focus has been shifted back to machine learning. Now that the technology has caught up, and so much of our life is visible through the data we supply when we interact with the internet, these algorithms have a wealth of information to study.
We’ve moved to a point where everyday applications of algorithms have become commonplace, and now, a newer set of more sophisticated tools are being developed that go beyond what many people ever expected was possible.
But that’s the interesting thing about AI — it keeps exceeding the expectations of what we think is possible:
- In 1997, IBM’s Deep Blue beat the world chess champion.
- In 2011, IBM’s Watson won a real-life game of Jeopardy.
- In 2016, DeepMind’s AlphaGo beat the world Go champion.
- In 2023, OpenAI’s GPT-4 passed the bar and USMLE.
Not everyone has access to the technology behind Deep Blue, Watson or AlphaGo, but GPT-4 is available to anyone with an internet connection.
This access — this super bloom of practical applications made available to the general public — is why we are here having a new and exciting discussion about AI today.
The New Wave of AI Tools
There are two main categories of new AI tools that are dominating the majority of the AI conversation: natural language models (ChatGPT) and art diffusion models (Midjourney, DALL-E & Stable Diffusion).
The next two parts of this series focus on each of those categories, respectively.
But to prime the pump, so to speak, let’s give you a basic understanding and example of each.
Natural Language Models
OpenAI has really dominated the market when it comes to language processing. The launch of ChatGPT was the big moment when the masses started paying attention, but it was more of a user interface than it was a language model. GPT-3.5, the “program” that ChatGPT uses to think, had already been around, as had older models like GPT-3 and 2.
What ChatGPT did was give users an easy access point. Instead of going the previous route, which meant injecting prompts right into the request part of previous GPT models, ChatGPT implemented a text chat style UI. It also allowed the model to use the previous conversation as context for every new response, which made it feel more intuitive than a sandbox.
No more spoilers, though; you’ll have to read the next part of the series to learn more about language models.
Art Diffusion Models
There are three big players here that most AI enthusiasts talk about: Stable Diffusion, DALL-E 2, and Midjourney. All three of these options can be accessed relatively easily, but their art output and user interfaces differ substantially. If you want to know how, we will dive into the specific in part 3 of our AI series.
For now, just know that each machine has been trained differently, but similarly — they are called diffusion models because they work by taking artwork that is already in existence and slowly diffusing (or dropping the information in the image) until it is completely gone. Then it practices doing that same process in the other direction. After enough training, it learns how to generate generally useful and exciting art.
You probably have some questions about it, and that’s expected. We will be covering most of, if not all, those questions in this series.
On Your Way to Understanding AI
These next sections answer the big question you have: how can I use these technologies in my practice, and how will they change or benefit my business? So, if you are interested in learning those answers, keep reading. We know you won’t regret it.
Too Long? Here's the Short Version
TL;DR Artificial intelligence has been a buzzword for decades, but it's only in recent years that its practical applications have come to the forefront, thanks to the digitization of our lives and the vast amounts of data available for machine learning algorithms to process. Today, two main categories of AI tools dominate the conversation: natural language models and art diffusion models. As we continue to integrate AI into our daily lives, understanding its capabilities and potential applications becomes crucial.
TL;DR Artificial intelligence has been a buzzword for decades, but it's only in recent years that its practical applications have come to the forefront, thanks to the digitization of our lives and the vast amounts of data available for machine learning algorithms to process. Today, two main categories of AI tools dominate the conversation: natural language models and art diffusion models. As we continue to integrate AI into our daily lives, understanding its capabilities and potential applications becomes crucial.